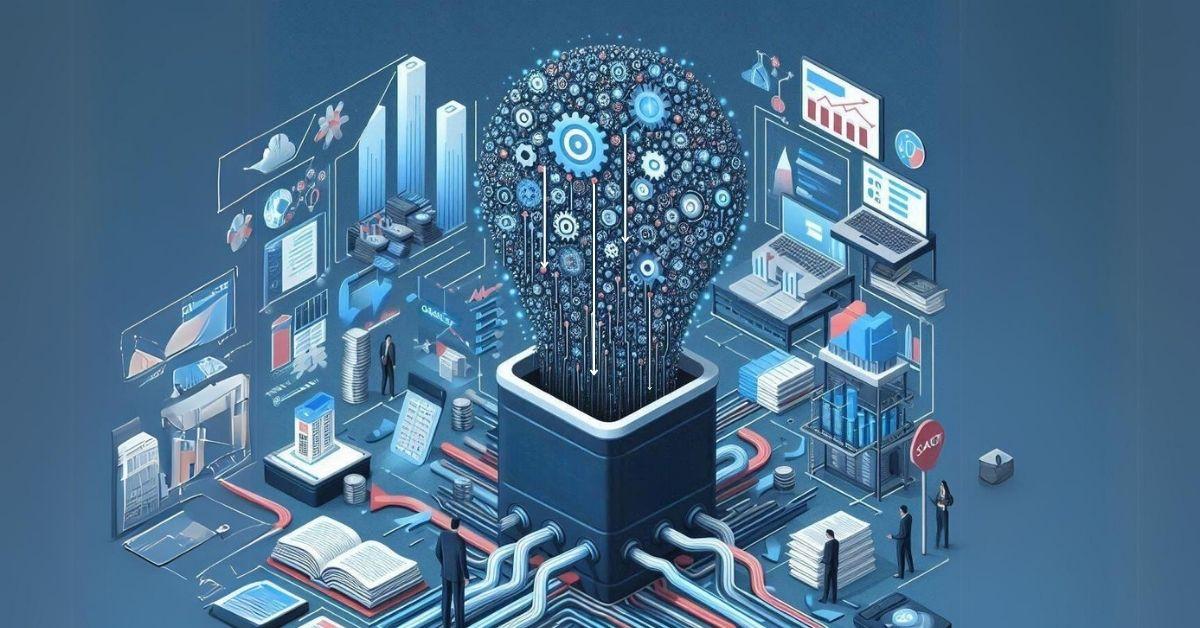
There is a new revolution in the finance industry and it is powered by generative AI. It is transforming how businesses approach accounting, financial planning and analysis (FP&A), compliance, and other aspects of how an enterprise operates.
This goes beyond traditional AI capabilities and allows for the creation of new data that can mimic existing datasets which can then power creative solutions for complex financial problems.
I am delighted share my thoughts about generative AI as a transformative technology, highlighting some applications, benefits, and case studies in the finance sector.
A game-changer: Generative AI in finance
It’s worth mentioning that generative AI differs from conventional AI as it can generate fresh, realistic data. The accuracy of such data is important, especially when it comes to the financial sector and its sub-domains.
Let’s see how generative AI changes key financial domains through actionable insights:
1. Accounting
Generative AI automates repetitive accounting processes, reduces human error rates, and enhances the accuracy of recorded information. Notably;
- Efficiency gains: Automating data entry, as well as reconciliation tasks, translates into a significant time savings for accountants, allowing them to focus on more valuable activities. Generative AI can streamline invoice processing, reducing the time spent on manual entries and reconciliations. That frees up the finance team to concentrate on strategic issues, such as budgets or pricing strategies.
- Greater accuracy: Artificial intelligence systems minimise errors in financial records, leading to more accurate accounts. For instance, there will be fewer differences between transaction logs and financial statements once they are cross checked by using generative AI tools. This enhances the accuracy of quarterly reports and means that stakeholders are far less likely to lose faith in a company due to mistakes made during these processes.
2. Financial Planning & Analysis (FP&A)
Generative AI changes FP&A through concepts like real-time dynamic forecasting as well as strategic decision making:
- Dynamic forecasting: AI models are capable of analysing massive amounts of data, including customer transaction data, market trends and macroeconomic factors in real-time. This, in turn, underpins more accurate and timely financial forecasts. Better forecast accuracy enables the swift adaptation to market changes and better informs investment decisions.
- Strategic decision-making: The provision of AI driven insights into financial information translates into well-developed long-term strategies and improved decision-making processes. By simulating various economic scenarios with generative AI, potential risks and opportunities can be better assessed, ultimately leading to more robust strategic development.
3. Compliance
AI plays a vital role in compliance checking by flagging suspicious transactions:
- Automated Monitoring: Financial transactions can be continuously monitored for regulatory compliance by AI systems, reducing anomalies in detection time during an audit process. This can improve AML (Anti-Money Laundering) compliance, allowing for proactive monitoring for suspicious activities, as opposed to the current approach of reactive monitoring. This is a significant potential gain as the current approach increases the likelihood of being penalised for non-compliance.
- Risk mitigation: Real-time identification of suspicious activities using AI reduces exposure to fraud risks and potential legal entanglements for firms. In addition, examining claim patterns and detecting fraudulent activities using generative AI tools can minimise the incidence of fraud through improvement on overall risk management procedures, providing more robust safety nets against such occurrences.
Real-world applications and benefits
Generative AI has made huge strides in finance having real gains across different domains:
1. Financial document analysis
- Natural language processing (NLP): Financial documents analysed by NLP algorithms, powered by artificial intelligence, allow for automated data entry, as well as extract insight from text. Contract reviews can be performed within a shorter time frame when automation is done through NPL. This results in better adherence to legal requirements while executing contracts or any other legal issues in a company.
2. Predictive analytics
- Predictive analytics: Artificial Intelligence models that use the previous financial data to predict future trends in investment plans and risk management are called predictive analytics. When it comes to customer data, the application of predictive analytics can ensure customised investment strategies, enhancing client satisfaction and customer retention.
- Anomaly detection: Using AI to detect irregularities in financial patterns helps organisations identify potential fraud and non-compliance issues. Fraudulent activities can be reduced given the rapid increase in accuracy in monitoring transactional patterns. This leads to greater customer trust in an enterprise.
3. Synthetic data generation
- Scenario simulation: For such implementations, GANs create synthetic datasets which replicate various financial scenarios, enabling enterprises to evaluate probable outcomes while developing responsive risk management procedures. Preparation for economic downturns is improved though stress testing and the modeling of different market conditions with synthetic data.
Real-world example 1:
Problem Statement
Manual invoice processing in accounting: Accounting departments of many non-tech companies like manufacturing still carry out slow and error-prone manual invoice processing.
Gen AI solution
The model will be trained by generating a synthetic invoice based on historical records to automate invoice processing using an AI system.
Implementation
Train a generative artificial intelligence (AI) model using the synthetic invoice generated information above. Here, invoices emanating through are processed with relevant details extracted such as supplier details, amounts and dates among others getting reconciled automatically with purchase orders and payment records.
Outcome
- Significantly reduced time spent on data entry
- Better efficiency when it comes to reconciliation
- The finance team gets space to go about tasks such a analysing financials or forecasting, thus creating value addition within them
Real-world example 2:
Problem statement
Inaccurate and slow financial forecasting in FP&A: Startups require more accurate and faster financial forecasts to make better decisions about investments.
Gen AI Solution
Real-time customer transactions’ analysis will be done alongside current market trends and macroeconomic indicators to produce dynamic predictions about the future state of things financially speaking.
Implementation
Introduce a generative AI model that will analyse the streams’ data continuously emanating from different sources. It gives forecasts within the current market environment by using accurate and timely generated predictions due to this.
Outcome
- Better accuracy of forecasts
- Reduced time spent on forecasts
- Facilitated rapid response to changing market conditions and informed investment decision-making
Real-world example 3:
Problem Statement
Challenges in compliance monitoring: Small regional banks experience inefficiencies while monitoring transactions for AML compliance which makes them overlook some suspicious activities putting them in regulatory risk.
Gen AI solution
Training of an AI model for real-time anomaly detection and compliance monitoring requires generating synthetic transaction data.
Implementation
Use synthetic transaction datasets to train generative AI. During this time, the system continuously monitors real-time transactions flagging any suspicious activities as well as alerting compliance officers for further action.
Outcome
- More accurate anomaly detection
- Faster compliance audit
- Ensured AML regulations compliant while reducing regulatory risks
Real-world example 4:
Problem statement
High volume of fraudulent insurance claims: The insurance industry loses huge amounts of money each year because it is unable to detect fraudulent claims early enough. Traditional methods are slow and often miss fraud entirely.
Gen AI Solution
Synthetic datasets will be created using historical claim records so as to train the AI model on fraud detection.
Implementation
Have a generative AI model trained with synthetic claim data. Incoming claims are analysed through this system, resulting in suspicious patterns being identified with potential fraud flagged for further review.
Outcome
- Fewer phony claims
- Enhanced overall risk management processes
- Quicker identification and resolution of fraudulent activities
Implementation Process
The successful implementation of generative AI in financial functions involves some key steps:
- Identifying key processes: Identify critical financial processes where integration with artificial intelligence (AI) can generate the highest efficiencies or improvements in regulatory requirements.
- Developing AI solutions: The selection should keep in mind the organisational protocols that must be adhered to during its deployment. Tailoring these models accordingly and in line with these specific processes will lead to optimal outcomes.
- Integration: Requires the joining of AI solutions to the existing financial systems to make them more efficient and seamless.
- Testing and validation: To guarantee the effectiveness and reliability in real-world applications, AI models have to be stringently tested.
Measuring Performance
It is extremely important to evaluate the performance of AI models in financial functions. Some of the key evaluation metrics include;
- Efficiency – how long it takes for data entry or financial analysis tasks to be completed
- Accuracy – improvement in accuracy levels of financial records and compliance reporting
- Compliance – ability to detect anomalies and adherence with regulatory standards
Conclusion
The accounting practices of the finance sector have been boosted by generative AI, such as FP&A transformation and compliance. It is one of the reasons why there are deep insights from new data, which are generated through this process. It is easier to have more efficient, accurate and strategic financial functions given how the technology has developed over time. AI solutions must be adapted in financial institutions so as to stay relevant in today’s complex world.
If a business puts a lot of emphasis on innovation, as well as utilises generative AI efficiently, it can lead to financial excellence. This, in turn, provides a greater opportunity for growing their business and succeeding in global markets.