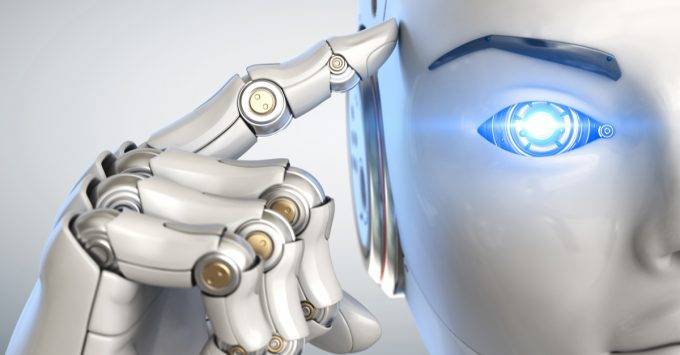
Last month on the FinTECHTalents blog, Award-winning insurtech company, Spixii, discussed AI and its potential ethical problems in risk modelling in AI and ethics: the ultimate Faustian bargain? If Artificial Intelligence (AI) became too effective at predicting who would make a claim, could it potentially outprice at-risk consumer groups? Here, Spixii explores the three features the insurance industry must consider to ensure AI better protects individuals and restores the trust to relationships between insurance companies and their customers.
Opening the black box
In ‘High Output Management’, Andrew Gove uses the “black box” analogy to describe a breakfast factory, where the raw material (eggs, bread and coffee) plus the labour of the waiters, managers and helpers goes in, and the output (the finished breakfast) comes out (Gove, 19). What happens in the middle?
Now, let’s take this analogy away from breakfast and towards AI in the insurance industry. Customer data and insurance expertise goes in and customer insights based on customer interaction comes out (in some cases, along with pricing and risk estimates). To transform this input into actionable insights, the algorithms and machine learning are the gears behind the black box.
It’s vital to see what these gears are doing and how they’re doing it to identify potential ethical and quality assurance purposes. If the AI is complex, it could be impossible or time-consuming to explain how it works – not only to a consumer but also a deep learning specialist. This has its consequences. In Part 1 of this blog post, we discussed the report that took a real-life example in healthcare, where the more complex model risked sending asthma sufferers with pneumonia home instead of to intensive care because of a quirk in the data: “It was hospital policy to send asthma sufferers with pneumonia to intensive care, and this policy worked so well that asthma sufferers almost never developed severe complications.” As the report states, “if there hadn’t been an interpretable model … “you could accidentally kill people” because of missing or incomplete data. In this case, the data was not due to negligence but hospital policy.
This is why it’s crucial to understand and interpret the gears of AI and machine learning. A clear, interpretable rule-based model is still preferable, so both companies and customers can see how the AI is generating insights and on what basis. This is also where modeller expertise and domain expertise must collaborate, and highlights the continuing importance of human knowledge.
A rule-based chatbot
The General Data Protection Regulation (GDPR) is fast approaching on the 25th of May 2018, plus the Insurance Distribution Directive (IDD). The IDD reinforces the importance of making chatbots compliant. You must be able to show customers understand what products they’re buying and why, which means companies must work even harder than before to protect individuals’ customer data and promote transparency.
So how can rule-based chatbots help?
Firstly, you can see how they work and approve every possible outcome. Natural language processing (NLP) is unable to accurately understand a user’s intention 100% of the time. When buying a product such as insurance or finance, NLP will need to be designed extremely well to handle the occasional misclassified user intents while meeting compliance.
Rebuilding trust
Arguably, AI can go one or two ways. On the one hand, it can make insurance easier to understand and help more people get better protected, through helping companies understand who their customers are, how best to engage them and ultimately rebuild trust in insurance. Or, customers will mistrust the AI when they don’t know what the algorithm does or what data it uses.
Alberto Chierici, co-founder and Chief Product Officer of Spixii, is a part-qualified actuary who’s used to considering the risk of new technologies. He’s passionate about considering ethical implications right at the beginning, before using technology. “I strongly believe if there are any ethical considerations to make, you cannot add them later. You cannot exploit technology and then try later to fix what went wrong,” he argues. “But, if the ethics are well-established, it is possible for AI to make insurance transparent and personal.”
“If you can communicate how it works to your customers – not the detailed equations but the general concept – and give a reason for the customer, this will help rebuild trust. For instance, let’s take what we do at Spixii: we may help an insurer to design and deploy an AI powered chatbot that offers personalised financial advice. For that, we would need to use some customer data to understand which affinity these customers belong to, and how they prefer to interact. Trust is all about starting the relationship with transparency and ethics rather than introducing it after.”
Ultimately, insurers and insurtechs alike have a duty to promote trust in new technology, AI and machine learning. If we’re truly going to shake some feathers in the industry and enhance digital customer experience, we must first carefully consider the impact of this technology and become advocates for ensuring its transparency.
To find out more about Spixii, please follow us on LinkedIn or get in touch with the Spixiifiers at team@spixii.ai